Studying new medicines and their properties is made that much easier thanks to supercomputers and artificial intelligence. Quantum computing is an ever-increasing reality. Experts believe that AI has democratized the development of medicines, helping to better understand chemistry while questioning certain work methods.
In 2021, the first drug developed using artificial intelligence was confirmed—a treatment aimed at patients with the rare condition of Huntington's disease. Developing new pharmaceuticals or identifying new uses for existing drugs is a complex process. How do supercomputing, machine learning, artificial intelligence, and quantum computing contribute to the discovery of new drugs?
It is estimated that there are approximately 10^60 pharmacological combinations possible with known compounds, and testing all of them becomes impractical every time a disease or ailment is under investigation.
“In silico techniques (meaning computer simulations, silico comes from the word silico, a basic component of classic computing) enable a pre-filtering of known substances”, explains Paulino Gómez-Puertas, co-ordinator of the Molecular Modelling Team at the Severo Ochoa Molecular Biology Centre (a mixed CSIC-UAM centre) in Madrid, by using supercomputing. “Instead of the millions of initial compounds, 3D computational simulation works like a sieve, selecting dozens of compounds for in vitro (test tube) or in vivo (in animals and even people) testing.“
“Artificial intelligence and machine learning techniques such as deep learning are being used in all stages of drug development,” says Nuria E. Campillo, CSIC research scientist, science communicator, and entrepreneur, noting that "it's an exceptionally lengthy, costly, and intricate process with a particularly high rate of failure". This technology assists in cutting costs and broadening accessibility for small and medium-sized enterprises (SMEs) and startups.
What do these new approaches contribute? "The main feature of machine learning is that the system is able to learn heuristically, meaning from examples, rather than through explicit instructions", states Alejandro Pazos Sierra, who holds a PhD in Medicine from the Complutense University of Madrid (UCM) and a PhD in Computer Engineering from the Polytechnic University of Madrid. He points out two particularly interesting characteristics of this approach: it comes up with solutions to complex problems and has the capacity to learn and adapt.
The power of AI when dealing with small scales
Artificial intelligence, as pointed out by Nuria Campillo, enables the design of entirely new molecules and the prediction of their properties. “There is enormous room for new molecules [potential combinations]. What we used to do with classic tools was modify existing molecules”. These were trapped in very compact clusters. But now the whole spectrum has been thrown wide open.
Albeit with certain limitations, of course. AI, Paulino Gómez-Puertas explains, searches for new drugs that would fit well into the cavity (or active site) of a certain enzyme. Thus, instead of relying on vast databases of existing compounds, it designs drugs that do not naturally occur. However, there is sometimes a technical issue: the theoretically proposed solution may be impossible to synthesize or prohibitively expensive. “You’re left wondering if it would have really worked or if it's what we call in technical-speak, a false positive, because you can’t manufacture it, which precludes it being tried out”.
Another challenge associated with AI systems is their black box nature. As Alejandro Pazos Sierra points out, quite often “it is unable to explain why it gets the results it does”. There are some fields, such as medical diagnosis for example, where this is critical. Machine learning, he adds, can be used "to identify potential drug molecules based on the molecular and structural shapes of target proteins".
"Enzymes [involved in certain diseases, like those granting antibiotic resistance to bacteria or helping viruses replicate], can be thought of as three-dimensional spheres with a cavity. This cavity is known as the active site, elaborates Gómez-Puertas, and the strategy involves searching for chemical compounds that can fill this space. and, thereby, inhibit the enzyme's function – whether it's breaking down antibiotics in bacteria or enhancing viral infections.
Artificial intelligence helps us to better understand chemistry
In 2023, the BBVA Foundation Frontiers of Knowledge Award in Biomedicine was presented for "advancements in the use of artificial intelligence (AI) to accurately predict the three-dimensional structures of proteins", accomplished by projects such as AlphaFold (AI and ML for protein folding) and Rosetta@home (a distributed computing project).
The fact that artificial intelligence and machine learning help us to better understand chemistry, and even to question some of the working methods and limited categories of ‘humans’ (e.g. is a virus a living organism?), is going to be particularly useful when it comes to tackling the appearance of super-bacteria or repositioning drugs.
Drug repurposing is straightforward—it involves discovering beneficial secondary effects of drugs that are already on the market, which translates to savings in research, testing, and approval costs. The capacity of super-bacteria is a bit harder to grasp: Paulino Gómez-Puertas explains it as follows: “Resistance to antibiotics normally occurs within a segment of DNA called a plasmid, which are found inside bacteria. Bacteria can physically transmit the plasmid between different species”. This represents a big challenge for hospitals.
The future, our best investment
Predict properties
In 2018, Atom2Vec artificial intelligence managed to recreate the periodic table of elements in two hours from a big molecule database. It has taken humans a century to understand how to order this information. According to a press release from Stanford University, AI might help us “discover new laws of nature.”
At present, we have reached a fascinating point: the predictive capability for properties of molecules we haven’t yet experimented with, thanks to AI's ability to learn from an immense amount of data. In pharmacology, predictability is crucial, and "being able to foresee whether a molecule will be toxic or well-absorbed is extremely beneficial", emphasizes Nuria Campillo, who also highlights the significant cost savings involved. “Changing a molecule after a start has been made on a research project is very expensive”.
Currently, Nuria Campillo and her company, AItenea Biotech, are engaged in designing and optimizing molecules and their properties using limited datasets. This has been made possible by the development of algorithms that require minimal data to train (similar to how a child learns what a cat is from just one or two examples). one or two examples suffice).
The next stage on the search for medicines will come with the aid of quantum computing. As the experience curve cheapens the cost of new methods owing to their massification, their use becomes more widespread. Let’s have a look at some stand-out examples:
- Photovoltaic. In 1975, it cost over USD 115 to generate 1 watt, whereas by 2020 it cost around USD 0.27.
- Analysis of the genome. Sequencing the genome cost USD 1 billion in 2000, but by 2015 it could be done at a cost of USD 1,000.
- Digital storage. Storing 1 GB of data in 1980 cost over half a million dollars, a cost which had dropped to USD 0.02 in 2020.
“AI has democratized the development of medicines and has enabled small companies to come on board in their development”, concludes Campillo. When quantum computer algorithms are developed and the quantum computing market stabilizes, there is no doubt use of these tools will become widespread among researchers.
Chart of US artificial intelligence in the health market (2020-2030)
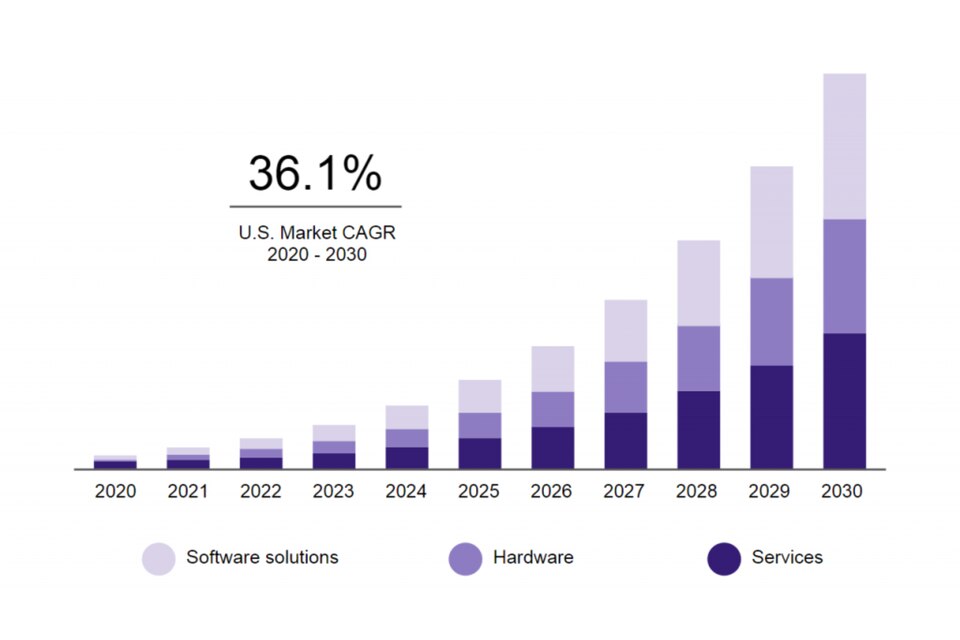